It is essential to determine the AI stock trading prediction's capability to adjust to changing market conditions since financial markets are dynamic, affected by policy changes and economic cycles. Here are 10 suggestions for assessing the model's capacity to adapt to market volatility.
1. Examine Model Retraining Frequency
Why is it that regular retraining makes sure that the model adapts to the latest data and changing market conditions.
What to do: Determine if the model is equipped with mechanisms for periodic training using current data. Models that go through periodic retraining will more likely to incorporate the latest trends or shifts.
2. Assess the use of adaptive algorithms
What's the reason? Certain algorithms, like reinforcement learning, or online models of learning are able to adapt to changes in patterns better.
How do you determine whether the model uses adaptive algorithms that are designed to adapt to changing environments. Algorithms that can adapt to the changing dynamics of markets include Bayesian networks and the recurrent network with adaptive learning rates.
3. Examine for the incorporation of the Regime Detection
Why: Different market conditions (e.g. bull, bear and high volatility) could affect the performance of an asset.
How: To identify the current market conditions and adjust its strategy, check if there are any detecting methods in the model such as concealed Markov or clustering models.
4. How can you assess the sensitivity to Economic Indices
Why: Economic factors, like inflation, interest and employment statistics can have a significant impact on stock market performance.
How do you determine if the model uses important macroeconomic indicators to permit it to recognize and respond to the larger economic changes that impact the market.
5. Examine how this model copes with volatile markets
The reason: Models that are unable to adapt to fluctuation will fail during volatile times or cause significant losses.
Analyze previous performance in turbulent periods. You can look for features that allow the model to be calibrated during periods of volatility, such as dynamic risks adjustment or volatility focusing.
6. Check for built-in drift detection mechanisms
Why: When certain statistical properties are altered in market data, it can influence the model's predictions.
How do you confirm that the model monitors and corrects any drift. Changepoint detection or drift detection may alert models to significant changes.
7. Assessing Features' Flexibility Engineering
The reason: Features that are rigid could become outdated due to market changes and reduce model accuracy.
How to: Look at adaptive feature engineering. This permits features in the model to be adapted to the current market conditions. A dynamic feature selection or periodic evaluation of features could increase the adaptability.
8. Examine the model's robustness in different types of assets
The reason is that if an algorithm is trained for just one asset class (e.g., equities) it could be unable to perform when it is applied to other classes (like bonds or commodities) which behave differently.
Test it out on various asset sectors or classes to determine how adaptable it can be. Models that can perform well across sectors and asset classes are more likely to be adaptable.
9. Think about hybrid or Ensemble models for greater flexibility
Why: Ensembles of models blend the theories of various algorithms to mitigate their weaknesses and enable them to adapt better to the changing environment.
How: Determine whether the model is based on an ensemble approach, such as combining trend-following and mean-reversion. Hybrid or ensemble models can alter strategies based on the market conditions, thereby increasing adaptability.
Review the Real-World Performance of Major Market Events
The reason: Testing the model in real-world situations will reveal its ability to adapt and resilience.
How to assess the historical performance in the event of major market disruptions. For these periods you can examine transparent performance data to determine the performance of the model and the extent to which its performance affected.
Concentrating on these points can assist you in assessing the adaptability of a stock trading AI predictor and ensure that it is robust under changing market conditions. This flexibility is essential in reducing risk and increasing the reliability of predictions across different economic conditions. View the top rated visit this link on artificial technology stocks for more tips including top ai stocks, cheap ai stocks, stocks for ai, best ai stocks, ai investing, invest in ai stocks, stock market prediction ai, ai on stock market, best site for stock, ai stocks to buy now and more.
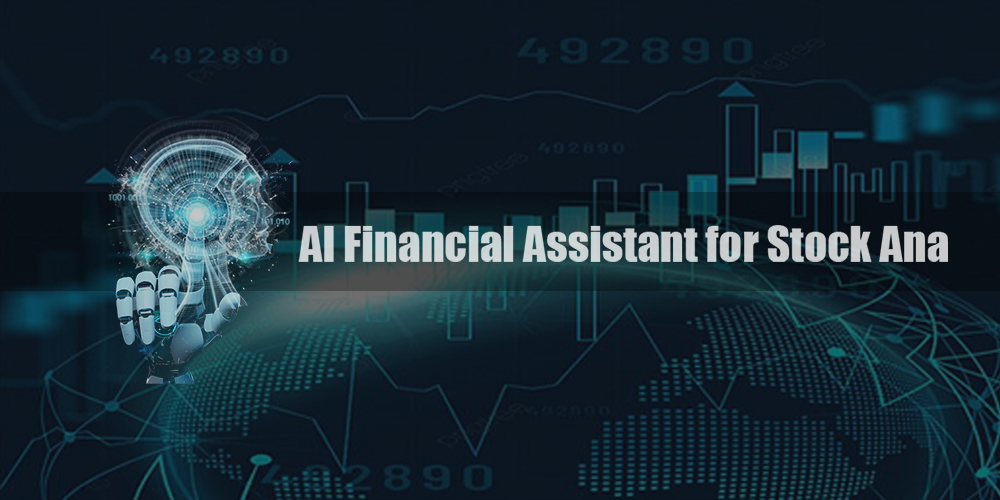
Top 10 Tips For Using An Ai Stock Trade Predictor To Determine The Amazon Stock Index
Understanding the business model and market dynamics of Amazon, along with economic factors that influence its performance, is essential to evaluating Amazon's stock. Here are ten tips to effectively evaluate Amazon’s stock using an AI-based trading model.
1. Understanding Amazon Business Segments
Why? Amazon operates across a range of industries, including streaming, advertising, cloud computing and e-commerce.
How: Familiarize yourself with the revenue contributions from each segment. Understanding the drivers of growth in these sectors helps the AI model to predict the overall stock performance, based on sector-specific trends.
2. Integrate Industry Trends and Competitor Analyses
Why: Amazon's performance is closely linked to changes in e-commerce, technology, and cloud-based services, and competitors from companies such as Walmart and Microsoft.
What should you do to ensure that the AI model can discern trends in the market, including online shopping growth rates and cloud adoption rates and changes in consumer behavior. Include the performance of competitors and market share analysis to provide context for Amazon's stock movements.
3. Earnings Reports Impact Evaluation
The reason: Earnings reports could result in significant price fluctuations, especially for high-growth companies like Amazon.
How: Monitor Amazon’s quarterly earnings calendar to see the way that previous earnings surprises have impacted the stock's performance. Include analyst and company expectations into your model to determine the future revenue forecasts.
4. Use Technical Analysis Indices
What are they? Technical indicators can be useful in finding trends and possible reverses in price movements.
How do you incorporate important technical indicators like moving averages, Relative Strength Index (RSI) and MACD (Moving Average Convergence Divergence) into the AI model. These indicators can be used to identify the most profitable starting and ending points in trades.
5. Analysis of macroeconomic factors
Why: Amazon's profitability and sales may be affected by economic conditions such as inflation, interest rates and consumer spending.
What should you do: Ensure that the model contains relevant macroeconomic indicators like consumer confidence indexes as well as retail sales. Knowing these variables improves the predictability of the model.
6. Implement Sentiment Analysis
Why: Stock prices can be influenced by market sentiments, particularly for companies that have a strong focus on consumers like Amazon.
How to use sentiment analysis of social media, headlines about financial news, and feedback from customers to determine the public's opinion about Amazon. By incorporating sentiment measurement, you can add valuable information to your predictions.
7. Be aware of changes to policies and regulations
Amazon's operations might be affected by antitrust regulations and privacy laws.
How do you keep track of policy developments and legal challenges related to e-commerce and technology. Make sure the model takes into account these variables to forecast possible impacts on Amazon's business.
8. Do backtests of historical data
The reason is that backtesting is used to assess how an AI model would perform if historical data on prices and events were used.
How to: Backtest predictions by using data from the past in Amazon's stock. Compare the model's predictions with the actual results to evaluate its reliability and accuracy.
9. Measuring Real-Time Execution Metrics
How to achieve efficient trade execution is critical to maximize profits, particularly with a stock as dynamic as Amazon.
What are the key metrics to monitor like slippage and fill rate. Evaluate how well the AI model is able to predict the optimal entry and exit points for Amazon trades, making sure that the execution is in line with the predictions.
Review the Risk Management and Position Size Strategies
The reason: Effective risk management is essential for capital protection, particularly in volatile stocks such as Amazon.
How: Make sure that the model includes strategies to manage the risk and to size your positions according to Amazon's volatility, as and your risk in the portfolio. This will help you reduce losses and maximize returns.
By following these tips, you can effectively assess the AI prediction tool for trading stocks' ability to understand and forecast movements in Amazon's stock, ensuring it remains accurate and relevant to changing market conditions. Take a look at the top rated stock market today advice for site recommendations including publicly traded ai companies, stock pick, top artificial intelligence stocks, cheap ai stocks, artificial intelligence and stock trading, ai share trading, chat gpt stock, ai and the stock market, best site to analyse stocks, artificial intelligence stock price today and more.